使用java + selenium + OpenCV破解網易易盾滑動驗證碼的示例
網易易盾:dun.163.com
* 驗證碼地址:https://dun.163.com/trial/jigsaw * 使用OpenCv模板匹配 * Java + Selenium + OpenCV
產品樣例
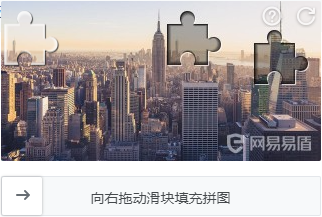
接下來就是見證奇跡的時刻!
註意!!!
· 在模擬滑動時不能按照相同速度或者過快的速度滑動,需要向人滑動時一樣先快後慢,這樣才不容易被識別。
模擬滑動代碼↓↓↓
/** * 模擬人工移動 * @param driver * @param element頁面滑塊 * @param distance需要移動距離 */ public static void move(WebDriver driver, WebElement element, int distance) throws InterruptedException { int randomTime = 0; if (distance > 90) { randomTime = 250; } else if (distance > 80 && distance <= 90) { randomTime = 150; } List<Integer> track = getMoveTrack(distance - 2); int moveY = 1; try { Actions actions = new Actions(driver); actions.clickAndHold(element).perform(); Thread.sleep(200); for (int i = 0; i < track.size(); i++) { actions.moveByOffset(track.get(i), moveY).perform(); Thread.sleep(new Random().nextInt(300) + randomTime); } Thread.sleep(200); actions.release(element).perform(); } catch (Exception e) { e.printStackTrace(); } } /** * 根據距離獲取滑動軌跡 * @param distance需要移動的距離 * @return */ public static List<Integer> getMoveTrack(int distance) { List<Integer> track = new ArrayList<>();// 移動軌跡 Random random = new Random(); int current = 0;// 已經移動的距離 int mid = (int) distance * 4 / 5;// 減速閾值 int a = 0; int move = 0;// 每次循環移動的距離 while (true) { a = random.nextInt(10); if (current <= mid) { move += a;// 不斷加速 } else { move -= a; } if ((current + move) < distance) { track.add(move); } else { track.add(distance - current); break; } current += move; } return track; }
操作過程
/** * 獲取網易驗證滑動距離 * * @return */ public static String dllPath = "C://chrome//opencv_java440.dll"; public double getDistance(String bUrl, String sUrl) { System.load(dllPath); File bFile = new File("C:/EasyDun_b.png"); File sFile = new File("C:/EasyDun_s.png"); try { FileUtils.copyURLToFile(new URL(bUrl), bFile); FileUtils.copyURLToFile(new URL(sUrl), sFile); BufferedImage bgBI = ImageIO.read(bFile); BufferedImage sBI = ImageIO.read(sFile); // 裁剪 cropImage(bgBI, sBI, bFile, sFile); Mat s_mat = Imgcodecs.imread(sFile.getPath()); Mat b_mat = Imgcodecs.imread(bFile.getPath()); //陰影部分為黑底時需要轉灰度和二值化,為白底時不需要 // 轉灰度圖像 Mat s_newMat = new Mat(); Imgproc.cvtColor(s_mat, s_newMat, Imgproc.COLOR_BGR2GRAY); // 二值化圖像 binaryzation(s_newMat); Imgcodecs.imwrite(sFile.getPath(), s_newMat); int result_rows = b_mat.rows() - s_mat.rows() + 1; int result_cols = b_mat.cols() - s_mat.cols() + 1; Mat g_result = new Mat(result_rows, result_cols, CvType.CV_32FC1); Imgproc.matchTemplate(b_mat, s_mat, g_result, Imgproc.TM_SQDIFF); // 歸一化平方差匹配法TM_SQDIFF 相關系數匹配法TM_CCOEFF Core.normalize(g_result, g_result, 0, 1, Core.NORM_MINMAX, -1, new Mat()); Point matchLocation = new Point(); MinMaxLocResult mmlr = Core.minMaxLoc(g_result); matchLocation = mmlr.maxLoc; // 此處使用maxLoc還是minLoc取決於使用的匹配算法 Imgproc.rectangle(b_mat, matchLocation, new Point(matchLocation.x + s_mat.cols(), matchLocation.y + s_mat.rows()), new Scalar(0, 255, 0, 0)); Imgcodecs.imwrite(bFile.getPath(), b_mat); return matchLocation.x + s_mat.cols() - sBI.getWidth() + 12; } catch (Throwable e) { e.printStackTrace(); return 0; } finally { bFile.delete(); sFile.delete(); } } /** * 圖片亮度調整 * * @param image * @param param * @throws IOException */ public void bloding(BufferedImage image, int param) throws IOException { if (image == null) { return; } else { int rgb, R, G, B; for (int i = 0; i < image.getWidth(); i++) { for (int j = 0; j < image.getHeight(); j++) { rgb = image.getRGB(i, j); R = ((rgb >> 16) & 0xff) - param; G = ((rgb >> 8) & 0xff) - param; B = (rgb & 0xff) - param; rgb = ((clamp(255) & 0xff) << 24) | ((clamp(R) & 0xff) << 16) | ((clamp(G) & 0xff) << 8) | ((clamp(B) & 0xff)); image.setRGB(i, j, rgb); } } } } // 判斷a,r,g,b值,大於256返回256,小於0則返回0,0到256之間則直接返回原始值 private int clamp(int rgb) { if (rgb > 255) return 255; if (rgb < 0) return 0; return rgb; } /** * 生成半透明小圖並裁剪 * * @param image * @return */ private void cropImage(BufferedImage bigImage, BufferedImage smallImage, File bigFile, File smallFile) { int y = 0; int h_ = 0; try { // 2 生成半透明圖片 bloding(bigImage, 75); for (int w = 0; w < smallImage.getWidth(); w++) { for (int h = smallImage.getHeight() - 2; h >= 0; h--) { int rgb = smallImage.getRGB(w, h); int A = (rgb & 0xFF000000) >>> 24; if (A >= 100) { rgb = (127 << 24) | (rgb & 0x00ffffff); smallImage.setRGB(w, h, rgb); } } } for (int h = 1; h < smallImage.getHeight(); h++) { for (int w = 1; w < smallImage.getWidth(); w++) { int rgb = smallImage.getRGB(w, h); int A = (rgb & 0xFF000000) >>> 24; if (A > 0) { if (y == 0) y = h; h_ = h - y; break; } } } smallImage = smallImage.getSubimage(0, y, smallImage.getWidth(), h_); bigImage = bigImage.getSubimage(0, y, bigImage.getWidth(), h_); ImageIO.write(bigImage, "png", bigFile); ImageIO.write(smallImage, "png", smallFile); } catch (Throwable e) { System.out.println(e.toString()); } } /** * * @param mat * 二值化圖像 */ public static void binaryzation(Mat mat) { int BLACK = 0; int WHITE = 255; int ucThre = 0, ucThre_new = 127; int nBack_count, nData_count; int nBack_sum, nData_sum; int nValue; int i, j; int width = mat.width(), height = mat.height(); // 尋找最佳的闕值 while (ucThre != ucThre_new) { nBack_sum = nData_sum = 0; nBack_count = nData_count = 0; for (j = 0; j < height; ++j) { for (i = 0; i < width; i++) { nValue = (int) mat.get(j, i)[0]; if (nValue > ucThre_new) { nBack_sum += nValue; nBack_count++; } else { nData_sum += nValue; nData_count++; } } } nBack_sum = nBack_sum / nBack_count; nData_sum = nData_sum / nData_count; ucThre = ucThre_new; ucThre_new = (nBack_sum + nData_sum) / 2; } // 二值化處理 int nBlack = 0; int nWhite = 0; for (j = 0; j < height; ++j) { for (i = 0; i < width; ++i) { nValue = (int) mat.get(j, i)[0]; if (nValue > ucThre_new) { mat.put(j, i, WHITE); nWhite++; } else { mat.put(j, i, BLACK); nBlack++; } } } // 確保白底黑字 if (nBlack > nWhite) { for (j = 0; j < height; ++j) { for (i = 0; i < width; ++i) { nValue = (int) (mat.get(j, i)[0]); if (nValue == 0) { mat.put(j, i, WHITE); } else { mat.put(j, i, BLACK); } } } } } // 延時加載 private static WebElement waitWebElement(WebDriver driver, By by, int count) throws Exception { WebElement webElement = null; boolean isWait = false; for (int k = 0; k < count; k++) { try { webElement = driver.findElement(by); if (isWait) System.out.println(" ok!"); return webElement; } catch (org.openqa.selenium.NoSuchElementException ex) { isWait = true; if (k == 0) System.out.print("waitWebElement(" + by.toString() + ")"); else System.out.print("."); Thread.sleep(50); } } if (isWait) System.out.println(" outTime!"); return null; }
註意:有一個問題還沒有解決,還無法區分陰影部分是黑色還是白色。 因為兩種的情況不同,所以處理方式也不同。陰影部分為黑底時需要轉灰度和二值化,為白底時不需要。黑底使用歸一化平方差匹配算法 TM_SQDIFF ,而白底使用相關系數匹配算法 TM_CCOEFF。
到此這篇關於使用java + selenium + OpenCV破解網易易盾滑動驗證碼的文章就介紹到這瞭,更多相關java滑動驗證碼內容請搜索WalkonNet以前的文章或繼續瀏覽下面的相關文章希望大傢以後多多支持WalkonNet!
推薦閱讀:
- 利用Java+Selenium+OpenCV模擬實現網頁滑動驗證
- python自動化操作之動態驗證碼、滑動驗證碼的降噪和識別
- OpenCV結合selenium實現滑塊驗證碼
- Python Selenium破解滑塊驗證碼最新版(GEETEST95%以上通過率)
- 使用java + OpenCV破解頂象面積驗證碼的示例